Breaking News
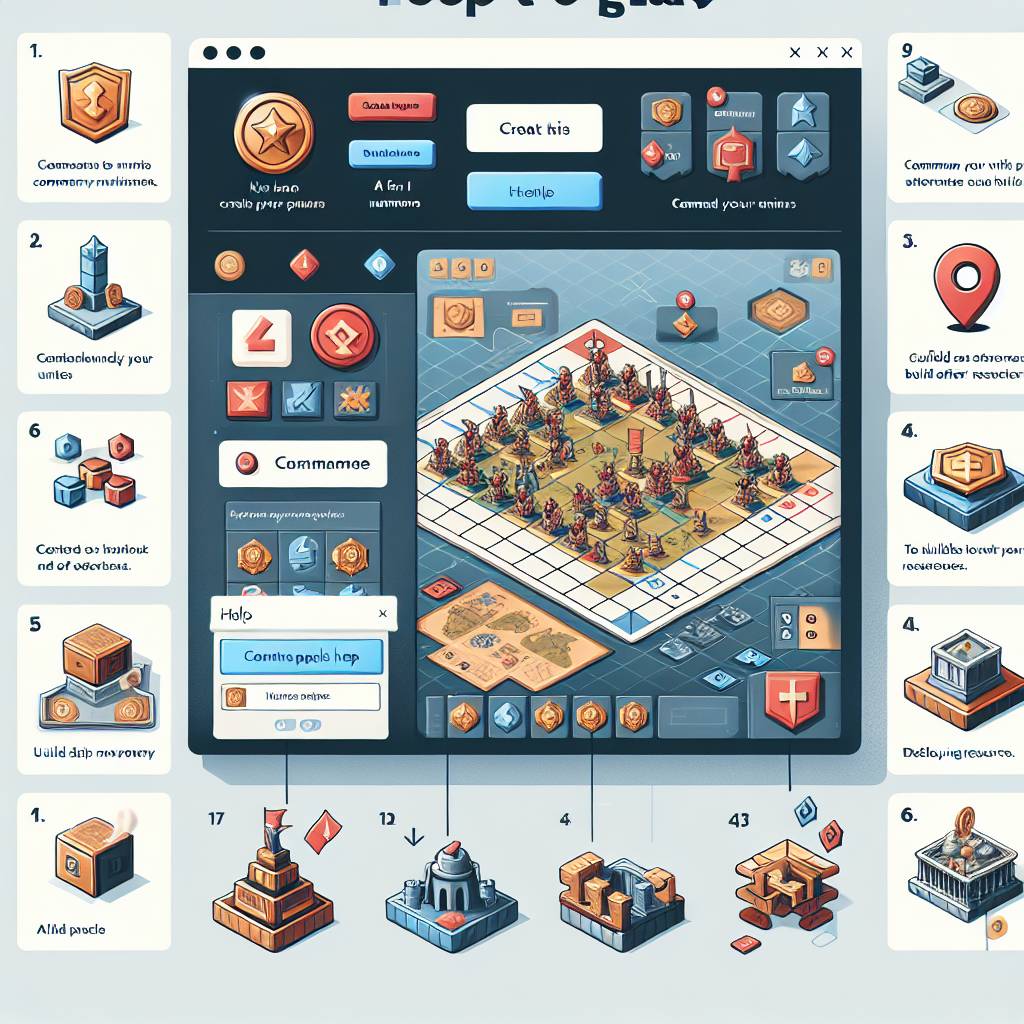
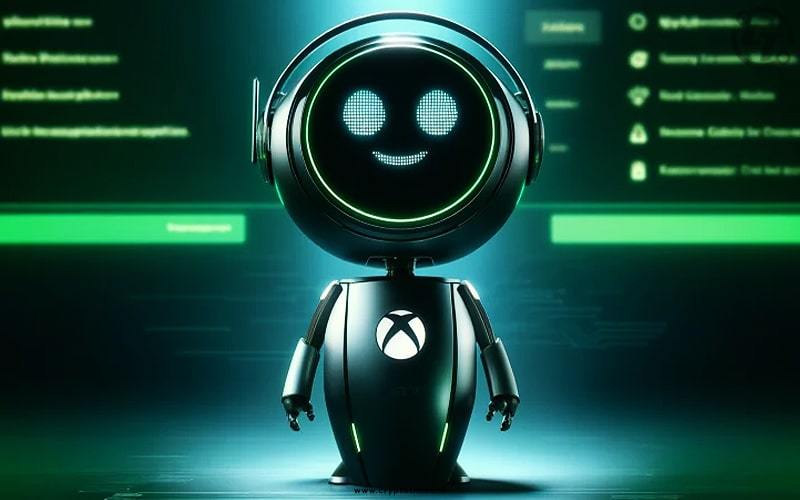
Popular News
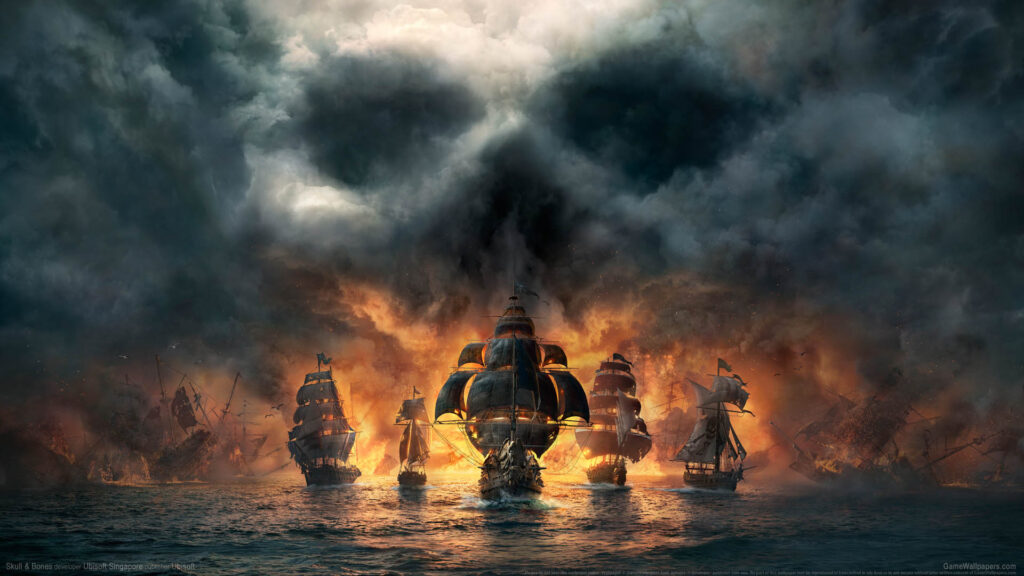
Discover how AI is revolutionizing site reliability engineering with predictive maintenance, automated incident resolution, resource optimization, anomaly detection, and continuous performance monitoring.Are you curious about the impact of artificial intelligence on site reliability engineering? In today’s technological landscape, AI-driven solutions are revolutionizing the way we approach and manage site reliability. From predicting and preventing potential issues with AI-driven predictive maintenance to automatically resolving incidents, optimizing resources intelligently, detecting anomalies in real-time, and continuously monitoring performance, AI is transforming the role of site reliability engineers.
In this blog post, we will explore the various ways in which AI is reshaping site reliability engineering practices. We will delve into the benefits of AI-driven predictive maintenance, automated incident resolution, intelligent resource optimization, real-time anomaly detection, and continuous performance monitoring, and showcase how these advancements are streamlining operations and improving the overall reliability of systems. Join us as we uncover the cutting-edge technologies and approaches that are driving the evolution of site reliability engineering through the power of AI.
Contents
AI-driven predictive maintenance is revolutionizing the way site reliability engineering (SRE) teams monitor and maintain systems. By harnessing the power of artificial intelligence, organizations can now predict when equipment might fail and take preemptive measures to prevent costly downtime. This proactive approach to maintenance not only improves the overall reliability of systems, but also reduces maintenance costs and extends the lifespan of equipment.
With the use of AI, SRE teams can analyze vast amounts of historical data to identify patterns and trends that may indicate potential issues. By utilizing machine learning algorithms, predictive maintenance models can be trained to recognize these patterns and provide early warnings for potential failures. This allows SRE teams to schedule maintenance at optimal times, reducing the need for costly emergency repairs and minimizing disruption to operations.
Furthermore, AI-driven predictive maintenance also enables organizations to shift from a time-based maintenance approach to a condition-based one. By continuously monitoring and analyzing real-time data from sensors and other sources, AI can identify anomalies and performance degradation, allowing for early intervention before a critical failure occurs. This shift not only improves the efficiency of maintenance operations, but also helps organizations make better use of their resources.
In conclusion, AI-driven predictive maintenance is a game changer for site reliability engineering, providing organizations with the ability to anticipate and prevent equipment failures before they happen. By leveraging artificial intelligence and machine learning, SRE teams can transform their approach to maintenance, reducing costs, improving reliability, and ultimately enhancing the overall performance of their systems.
In today’s fast-paced digital world, incidents and outages can occur at any time, causing major disruptions to businesses and their customers. Site reliability engineers are constantly under pressure to resolve these issues quickly and efficiently, to minimize the impact on the organization. This is where automated incident resolution comes into play, leveraging the power of AI to detect, diagnose, and resolve issues in real-time, without the need for human intervention.
With the help of AI-driven algorithms, automated incident resolution tools can analyze vast amounts of data from various sources to quickly identify the root cause of an issue and initiate the appropriate remediation actions. By automating the incident resolution process, organizations can significantly reduce the mean time to resolution (MTTR), thereby improving the overall reliability and availability of their digital services.
Furthermore, these AI-powered tools can learn from past incidents and continuously improve their accuracy and effectiveness in resolving future issues. By leveraging historical data and patterns, the automated incident resolution systems can proactively detect and prevent potential outages, ultimately enhancing the reliability and performance of the organization’s infrastructure.
Benefits of Automated Incident Resolution | Impact on Site Reliability Engineering |
---|---|
|
|
|
|
In conclusion, the adoption of automated incident resolution tools powered by AI is revolutionizing the field of site reliability engineering, enabling organizations to proactively manage and mitigate incidents, while continuously improving the reliability and performance of their digital infrastructure.
In the fast-paced world of site reliability engineering, the need for intelligent resource optimization has never been greater. With the increasing complexity of modern IT systems, companies are turning to AI-driven solutions to help them make the most out of their resources.
One of the key benefits of AI-driven resource optimization is the ability to analyze vast amounts of data in real time. This allows companies to identify patterns and trends that would be impossible to spot with traditional monitoring tools.
By leveraging the power of AI and machine learning, organizations can automatically adjust their resource allocation based on changing demands. This not only improves performance and reliability, but also helps reduce costs by ensuring that resources are used efficiently.
Benefits of Intelligent Resource Optimization |
---|
|
In the fast-paced world of site reliability engineering, it is crucial to have real-time anomaly detection in place to quickly identify and address any issues that may arise. With the advancement of AI-driven technology, companies can now leverage sophisticated algorithms and machine learning models to continuously monitor their systems for any sudden deviations from the norm. This not only allows for early detection of potentially harmful anomalies, but also provides the opportunity to proactively optimize system performance and prevent downtime.
By implementing automated incident resolution processes alongside intelligent resource optimization, organizations can ensure that any anomalies detected in real-time are promptly addressed without the need for manual intervention. This not only reduces the burden on human operators, but also minimizes the impact of anomalies on overall system stability and reliability.
Furthermore, continuous performance monitoring plays a key role in the effectiveness of real-time anomaly detection. By regularly collecting and analyzing data from various system components, organizations can gain valuable insights into their infrastructure’s behavior and identify potential anomalies before they escalate into critical issues. This proactive approach to anomaly detection and resolution is essential in maintaining a high level of system availability and performance.
Overall, the integration of AI-driven predictive maintenance and real-time anomaly detection is transforming the way organizations approach site reliability engineering. By harnessing the power of advanced technologies, companies can proactively monitor and optimize their systems, ensuring minimal disruption and maximum performance.
Continuous performance monitoring is a critical aspect of site reliability engineering, ensuring that the system is operating optimally at all times. With the advent of AI-driven technologies, performance monitoring has become more efficient and effective than ever before. AI algorithms can analyze vast amounts of data in real-time, identifying potential performance issues and anomalies before they escalate into major incidents.
Through automated incident resolution, AI can not only detect performance issues but also take proactive measures to resolve them. This helps in minimizing downtime and maintaining a seamless user experience. By leveraging AI for continuous performance monitoring, organizations can ensure that their systems are always running at peak efficiency, without the need for manual intervention.
Implementing intelligent resource optimization is another benefit of AI-driven continuous performance monitoring. By analyzing performance data and user patterns, AI can dynamically allocate resources to different parts of the system in real-time, ensuring optimal performance and resource utilization. This level of automation and optimization is simply not possible through traditional monitoring methods.
To illustrate the impact of AI in continuous performance monitoring, consider the following table:
Traditional Monitoring | AI-driven Monitoring |
---|---|
Reactive approach to performance issues | Proactive identification and resolution of issues |
Manual resource allocation and optimization | Dynamic and intelligent resource allocation |
High risk of system downtime and performance degradation | Minimal downtime and optimal system performance |
What is site reliability engineering (SRE)?
SRE is a discipline that incorporates aspects of software engineering and applies them to infrastructure and operations problems.
How is AI impacting SRE?
AI is transforming SRE by automating routine tasks, predicting and preventing outages, and optimizing system performance.
What are some examples of AI applications in SRE?
Examples include using AI for anomaly detection, capacity planning, and incident response in SRE.
How can AI improve reliability in engineering?
AI can improve reliability in engineering by identifying patterns, detecting abnormalities, and providing insights for proactive maintenance.
What are the benefits of integrating AI into SRE practices?
The benefits include faster incident resolution, increased system stability, and more efficient resource allocation.
Are there any challenges in implementing AI in SRE?
Challenges may include data quality issues, the need for specialized skills, and ensuring AI models are transparent and explainable.
What does the future hold for AI in SRE?
The future of AI in SRE includes advancements in machine learning, increased automation, and the potential for AI to become an integral part of SRE processes.