Breaking News
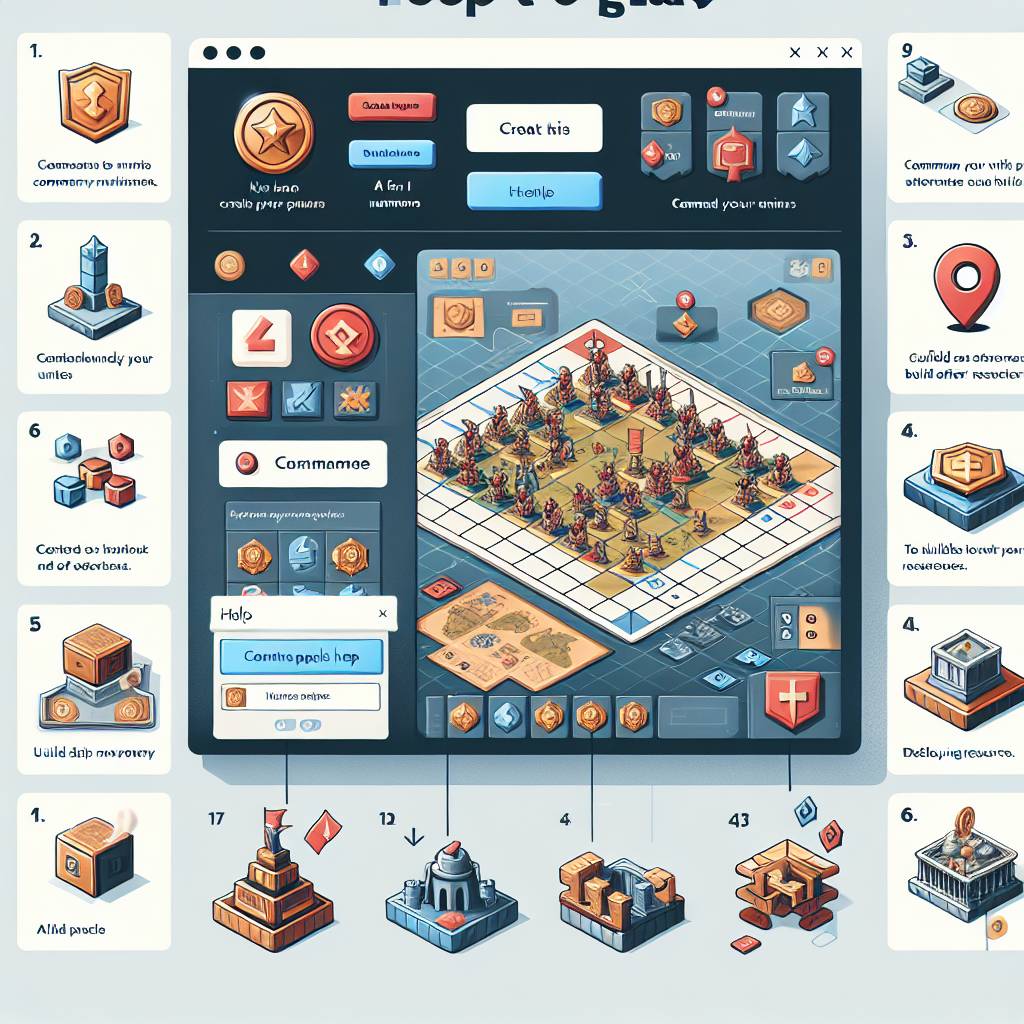
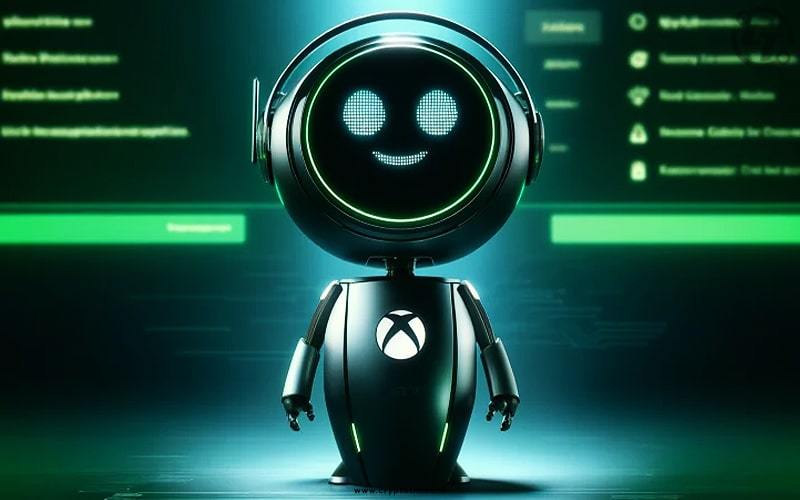
Popular News
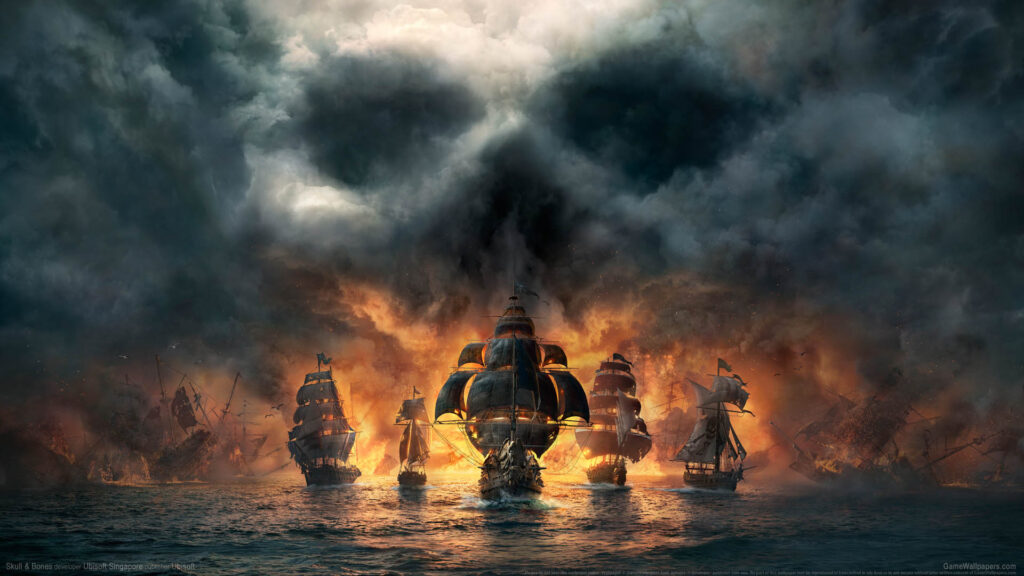
Artificial neural networks, driving breakthroughs in diverse fields from finance to healthcare, continue to be a puzzle due to their ‘black box’ nature. These networks, while powerful, often leave engineers and scientists struggling to comprehend their internal workings.
A team led by data and computer scientists at the University of California, San Diego has made significant strides in demystifying how artificial neural networks learn. They’ve developed a new formula that simplifies the understanding of how these networks identify and use patterns in data.
The researchers discovered that a statistical formula commonly used in analysis provides a simplified mathematical explanation of how neural networks, like the predecessor to ChatGPT, GPT-2, learn patterns in data. This revelation not only sheds light on how these networks learn but also how they make predictions.
The study’s lead author, Daniel Beaglehole, explains that this formula allows easier interpretation of the features networks use to make predictions. Published in Science Magazine on March 7, this research highlights the formula’s potential to revolutionize understanding and training of neural networks.
Despite their widespread use in approving bank loans, analyzing medical data in hospitals, and screening job applications in companies, understanding the decision-making process of neural networks and eliminating biases remains challenging. Professor Mikhail Belkin, another lead researcher, emphasizes the difficulty in ensuring that neural networks produce accurate and appropriate responses without a clear understanding of their learning mechanisms.
The concept of ‘feature learning’ is crucial, as it enables networks to recognize and use data patterns for making predictions. For instance, identifying whether a person in a photo is wearing glasses involves recognizing specific features, such as the top of the face or around the eyes and nose.
This study offers a significant step forward in understanding and potentially enhancing the performance of computational systems, including those not based on neural networks. By elucidating the selective attention mechanisms of neural networks, the researchers hope to pave the way for simpler, more efficient, and more understandable machine learning models, contributing to the broader adoption of artificial intelligence technology.